Artificial intelligence (AI) is increasingly being used in critical decision-making processes, from hiring employees to determining loan approvals and diagnosing medical conditions. However, AI systems are only as fair as the data they are trained on. If AI models are built on biased datasets, they can reinforce and even amplify societal inequalities, leading to unfair outcomes.
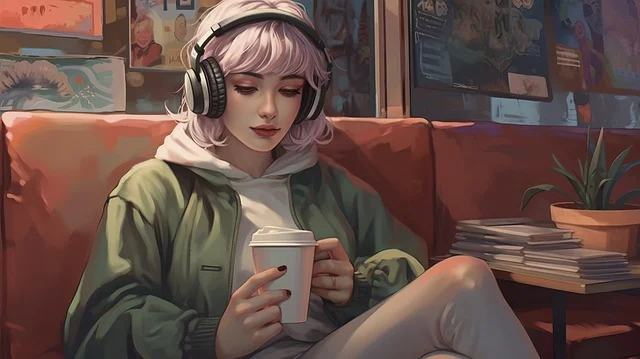
How Bias Creeps into AI
Bias in AI can stem from multiple sources, including:
1. Bias in Training Data
AI models learn patterns from historical data. If this data reflects existing prejudices, the AI will replicate them.
- Example: A hiring AI trained on past resumes may favor male candidates if previous hiring decisions showed gender bias.
2. Algorithmic Bias
Even with balanced data, the way an AI algorithm processes information can introduce bias. Certain features may be weighted unfairly, leading to skewed results.
- Example: An AI system for credit scoring may unintentionally favor wealthier neighborhoods, disadvantaging minority communities.
3. Bias in Data Collection
If data is not representative of diverse populations, AI models may be less accurate for underrepresented groups.
- Example: Facial recognition technology trained on predominantly white faces may struggle to correctly identify people of color.
1. Amazon’s AI Hiring Tool
In 2018, Amazon scrapped an AI hiring tool after discovering it discriminated against women. The system was trained on resumes submitted over the past decade, which were mostly from men. As a result, the AI favored male candidates and penalized resumes that included words like “women’s” (e.g., “women’s soccer team”).
2. Racial Bias in Facial Recognition
Studies from MIT and the ACLU have shown that facial recognition technology has higher error rates for people of color.
- In 2018, the American Civil Liberties Union (ACLU) tested Amazon’s Rekognition software, which falsely identified 28 U.S. Congress members as criminals. Most of those misidentified were people of color.
3. Healthcare Discrimination
A 2019 study found that an AI-driven healthcare risk assessment algorithm showed racial bias. It was more likely to assign lower risk scores to Black patients, resulting in fewer referrals for advanced care compared to white patients with similar health conditions.
Consequences of AI Bias
- Discrimination in Hiring: Biased AI tools can exclude qualified candidates based on gender, race, or other factors.
- Unfair Loan Approvals: AI-driven credit scoring may deny loans to certain groups based on historical data rather than individual financial behavior.
- Flawed Criminal Justice Decisions: AI-based risk assessment tools used in sentencing and parole decisions can disproportionately target marginalized communities.
- Healthcare Inequities: AI models that fail to consider diverse populations can lead to misdiagnoses and unequal access to treatment.
How to Reduce AI Bias
- Use Diverse and Representative Datasets
- AI developers must ensure datasets include a broad range of demographics to prevent underrepresentation.
- Regularly Audit AI Systems for Bias
- Organizations should test AI models to identify and correct biases before deployment.
- Implement Explainable AI (XAI)
- AI systems should provide transparent explanations for their decisions, allowing humans to detect bias.
- Establish Ethical AI Guidelines
- Governments and organizations should enforce fairness and accountability in AI development.
- Human Oversight in AI Decisions
- AI should assist, not replace, human judgment in critical areas like hiring, healthcare, and criminal justice.
Conclusion
Bias in AI is a significant ethical challenge that can lead to discrimination, inequality, and unfair decision-making. While AI has the potential to improve efficiency and accuracy, it must be designed with fairness in mind. By ensuring diverse datasets, conducting regular audits, and maintaining human oversight, we can develop AI systems that promote fairness and equality rather than reinforce existing biases.
Would you like me to add more details on AI bias mitigation strategies or real-world examples?