Artificial intelligence (AI) is transforming industries, but it also raises serious concerns about fairness and bias. AI systems are designed to make decisions based on data, but if that data reflects existing societal biases, the AI can reinforce and even worsen inequalities. Ensuring fairness in AI development is essential to prevent discrimination in areas such as hiring, finance, law enforcement, and healthcare.
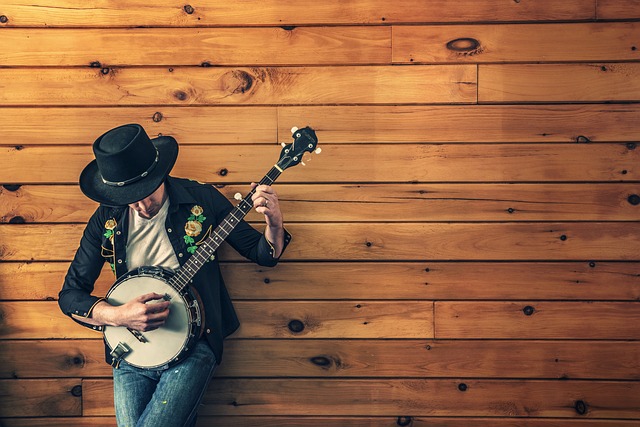
1. How Does Bias Enter AI Systems?
Bias in AI arises from multiple sources, including:
1.1 Bias in Training Data
AI learns from historical data, and if that data contains biases, the AI will inherit and amplify them.
- Example: If a hiring algorithm is trained on past job applications where men were predominantly hired, it may learn to favor male candidates over equally qualified women.
1.2 Algorithmic Bias
Even if the data is unbiased, the way an AI system processes it can introduce bias. Some algorithms weigh certain factors more heavily, unintentionally favoring or discriminating against particular groups.
- Example: AI-powered lending systems may assign lower credit scores to individuals from low-income neighborhoods, even if their financial behavior is responsible.
1.3 Bias in Data Collection
AI models perform best when they have diverse and representative datasets. If certain groups are underrepresented in the data, the AI may make incorrect or unfair predictions for those populations.
- Example: Facial recognition software trained mostly on white faces has been found to misidentify people of color at significantly higher rates.
2.1 Amazon’s AI Hiring Tool
Amazon developed an AI-powered hiring tool that was found to discriminate against female applicants. The AI had been trained on past hiring data, which was male-dominated. As a result, the system downgraded resumes that included words like “women’s,” such as “women’s soccer team.” Amazon eventually scrapped the tool due to its bias.
2.2 Racial Bias in Facial Recognition
A 2018 study by MIT found that facial recognition technology from major tech companies had much higher error rates when identifying women and people of color.
- The American Civil Liberties Union (ACLU) tested Amazon’s Rekognition software, which falsely identified 28 U.S. Congress members as criminals, with a disproportionate number of errors affecting Black and Latino members.
2.3 Healthcare Inequality
A 2019 study found that a widely used AI-driven healthcare risk prediction algorithm was biased against Black patients. The system was designed to prioritize high-risk patients for additional care, but it systematically underestimated the health risks of Black individuals compared to white patients with similar conditions.
When AI systems are biased, the impact can be severe:
- Hiring Discrimination: AI-driven recruitment tools may favor certain demographics, leading to fewer opportunities for underrepresented groups.
- Unfair Loan Approvals: AI-based credit scoring may reject applications from minorities or low-income individuals due to historical biases.
- Criminal Justice Issues: AI-powered predictive policing systems may disproportionately target certain communities, reinforcing systemic racism.
- Healthcare Disparities: AI-driven medical diagnostics may be less accurate for minority groups, leading to misdiagnosis and inadequate treatment.
4.1 Use Diverse and Representative Data
Developers should ensure that AI models are trained on datasets that include a variety of demographics, backgrounds, and experiences.
4.2 Regularly Audit AI Systems
AI models should be tested for bias before deployment. Companies and governments should conduct ongoing audits to ensure fairness.
4.3 Implement Explainable AI (XAI)
AI systems should provide clear, human-readable explanations for their decisions. This allows people to identify and correct biased reasoning.
4.4 Ethical AI Regulations
Governments and organizations should establish guidelines for AI fairness and hold developers accountable for biased systems.
4.5 Human Oversight
AI should not make final decisions in high-stakes situations like hiring, lending, or medical diagnoses. Humans should always have the ability to review and override AI decisions.
5. Conclusion
Bias in AI is a major ethical challenge that can reinforce discrimination and inequality. While AI has the potential to improve decision-making and efficiency, it must be developed and used responsibly. By prioritizing fairness, transparency, and accountability, we can build AI systems that promote equality rather than deepen existing biases.
Would you like additional details on bias mitigation strategies or other real-world examples?